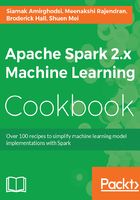
上QQ阅读APP看书,第一时间看更新
Performing matrix arithmetic using Spark 2.0
In this recipe, we explore matrix operations such as addition, transpose, and multiplication in Spark. The more complex operations such as inverse, SVD, and so on, will be covered in future sections. The native sparse and dense matrices for the Spark ML library provide multiplication operators so there is no need to convert to Breeze explicitly.
Matrices are the workhorses of distributed computing. ML features that are collected can be arranged in a matrix configuration and operated at scale. Many of the ML methods such as ALS (Alternating Least Square) and SVD (Singular Value Decomposition) rely on efficient matrix and vector operations to achieve large-scale machine learning and training.