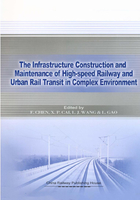
GEOLOGICAL HAZARD SUSCEPTIBILITY ASSESSMENT FOR RAILWAY NETWORK IN GUIZHOU PROVINCE
Rui TANG1,Weidong WANG1,2,Jie MA3,Yanping CHEN4
1School of Civil Engineering,Central South University,Changsha 410075
2National Engineering Laboratory for High Speed Railway Construction,Changsha 410075
3China Railway Eryuan Engineering Group Company Limited,Chengdu 610031
4China Railway Siyuan Survey and Design Group Company Limited,Wuhan 430063
Abstract:This paper took Guizhou Province as the study area,one of the places in China which are most seriously affected by landslide hazards.The research in this paper was conducted in three stages.Firstly,the landslide susceptibility mapping of railway was acquired by applying competition network model,and a set of conditioning factors were selected as the major landslide-conditioning factors,including elevation,lithology,rainfall,distance from river,distance from tectonic line,karst density and slope.Then,the concept of “degree of fitting” was proposed in the assessment of railway risk degree,and it was regarded as one of the three elements which determine the railway protection gradeon geological disasters.Finally,the matter-element model was established based on extension method,which can be used to evaluate the protection grades for the planned railway on geological disasters by integrating three elements,the train speed,grade of geological disaster susceptibility,and fitting degree between landslide inventory and railway line,into the model.
Keywords:geological disaster,landslide susceptibility,the planning railway,competition network,extension method,degree of fitting
Email:147745@163.com
1 Introduction
The research on geological disaster susceptibility mapping began in the 1960s.Current methods for geological disaster susceptibility mapping can be categorized into two groups,i.e.,qualitative and quantitative.Qualitative methods are mainly on the basis of some fuzzy approaches,combining with the experiences of the experts to make decisions,and quantitative methods generally rely on mathematical models and some software or data equations to calculate.
A considerable amount of research works have been conducted over the past years.In abroad,Varnes studied the type and movement processes of slope geological disasters,and put forward early analysis and control methods[1];Pistocchi established a landslide susceptibility map with expert system method,achieving good results[2];Yesilnacar utilized artificial neural network model and set up a landslide hazard susceptibility map for Turkish[3];Melchiorre studied the landslide sensitivity evaluation on the basis of neural network model and cluster analysis[4].In our country,many research methods about geological hazard susceptibility mapping have also been carried out in recent years.Yin at China University of Geosciences studied the alarm and forecast system of sudden geological disasters in Zhejiang Province[5];Dai summarized the various research methods on landslide disasters[6].
Guizhou Province in southwest China is taken as the project example in this paper,where landslides frequently occurred.According to the susceptibility degree on geological hazard and the railway network,this research obtained the potential areas wherethe railway safety was affected,and then assessed the protection grades for planning railway in Guizhou Province,in the hope of taking different measures to prevent disaster occurrence.
2 Geological Hazard Susceptibility Evaluations
2.1 Selection of landslide-causing factors
According to the geology,topography and other natural conditions,this paper comprehensive selected seven conditioning factors of elevation,lithology,rainfall,and distance from river,distance from tectonic line,karst density and slope used for determining the geological hazard susceptibility mapping.
In order to classify and quantify the hazard factors,this research divided them into several sub-classes.The area of history disaster within each sub-class was calculated and the area ratio between them was normalized.
2.2 Factor weight determination based on competition network
Competition network consists of a set of layers,namely,input layer,hidden layers,and output layer(Figure 1).
Each layer in the competition network consists of independent processing units called neurons.These neurons are linked to neurons in other layers through the weights and bias.

Figure 1 Architecture of competition network
The normalized data of the seven factors are regarded as the input layer,and the landslide risk degrees as the output layer.
By staking the seven factors together in AcrMap platform,the original layers of each factor were divided into fine and irregular polygons,which contained all normalized data of the seven factors.This paper took the whole Guizhou Province as the study area which was divided into 4073 small polygons,from which twelve typical polygons were selected as the test data.
After repeatedly adjusting the weight parameters,the factor weights used to make the 12 polygons to be classified accurately were acquired.Using these well-trained data,the whole dataset of Guizhou Province was imported and trained in one time based on the Matlab software.
2.3 Geological susceptibility map of Guizhou Province
After importing the Matlab calculating results into the DBF tables in ArcGIS and rendering different sensitivity degrees based on ArcMap,the landslide susceptibility map is obtained.

Figure 2 Landslide susceptibility map of Guizhou Province
According to the difference in landslide susceptibility degree,the study area is divided into five grades:the very low susceptible zone(VLS),the low susceptible zone(LS),the moderate susceptible zone(MS),the moderate-high susceptible zone(MHS)and the high susceptible zone(HS).
The statistics of the historical disaster area among each risk degree are shown in Table 1.
Table 1 Landslide distribution in landslide susceptible zones

3 Geological Hazard Assessment for Railway Network in Guizhou Province
According to the geological susceptibility map and historical landslide hazard distribution,we obtained the risk degrees along the planning railway line within Guizhou Province and got the distribution of landslide hazard points on both sides of railways(Figure 3).
According to the distribution proportion among five landslide risk degrees,each railway is endowed a hazard weight.The five grade weight coefficient θ=(7,5,3,2,1).For example,the hazard weight of HuKun passenger special line is:W1=wθT=(35.6%,25.4%,17.3%,18.9%,2.8%)·(7,5,3,2,1)T=4.69.

Figure 3 Planningrailways in Guizhou Province
The distribution ratio of the 21 planning railway lines in Guizhou Province among 5 landslide risk degrees is shown in Table 2.
Table 2 Distribution ratio and hazard weight of planning railway lines

3.1 Assessment of railway risk degree based on fitting degree
By using the concept of fitting degree[7-9],it is assumed that each railway line is a regression line(or curve),and the historical disaster points nearby the railway are the discrete points on both sides of the regression line.If the historical disaster points more densely distributes in the vicinity of the line,the fitting degree will be greater,and the risk of the railway will be higher.
This paper extracted all the planning railways in Guizhou Province,and took the buffer of 10 km on both sides of the railway lines for research based on ArcMap software(Figure 4).
The fitting degrees of each railway are calculated in the attribute Table 3.

Figure 4 Landslide hazard distribution within railway buffer of 10 km
Table 3 Fitting degree of planning railway lines

3.2 Railway protection grade assessment
Based on extension method,this paper selected three elements as the matter-element model:train speed,hazard weight and fitting degree.The specific steps are as follows[10-13].
3.2.1 Determining classical field
Table 4 lists the five protection grades according to the numerical characteristics of train speed,hazard weight and fitting degree,respectively.
Table 4 Factors affecting railway protection grades

3.2.2 Determining segment field
The segment field of the protection grade,namely the upper-lower limit of classical field,is expressed as:

3.2.3 Determining matter-element
Planning railway is expressed in the form of matter-element as:
HuKun passenger special line:

Gui-Zhu fast railway:

3.2.4 Determining connection degree between matter-elements and protection grade


aiand biare the upper or lower limit of Vti.
The connection function between ithelement and jthprotection grade is:

3.2.5 Determining weight coefficient
In this paper,λ1=λ2=λ3=1/3.
3.2.6 Calculating connection degree based on connection function and weight coefficient

Repeating Steps(4),(5),(6),the connection degree under other protection grade can be obtained.,the matter-element belongs to Kj0.The protection grades of the 21planning railways are shown in Table 5.
Table 5 Protection grades of each railway

It can be seen from Table 5 that the overall 21planning railways need moderate or high grade protection,and there are even many railroads need key protection such as the six railroads inⅤ protection grade and the four railroads in Ⅳprotection grade.
4 Results and Conclusions
(1)Competition network model is used to divide the landslide risk mapping into 5 grades.With the increase of risk degree,the distribution of the historical landslide disaster is denser,which indicates that the competition network model is effective and sensitive for the landslide susceptibility mapping.
(2)According to the distribution of landslide disaster points on both sides of railway,this paper puts forward the concept of “fitting degree”.The greater the fitting degree is,the closer the landslide disaster points are to the railway line,indicating that the risk of the railway is high,and the higher protection grade will be needed.
(3)Extension method is used to calculate the protection grade forplanning railway on geological disasters.As establishing the matter-element model,three elements are used:the train speed,fitting degree and the grade of geological disaster susceptibility.
Acknowledgement
The authors wish to acknowledge the support and motivation provided by Geological Hazard Susceptibility Mapping and Assessment in Basaltic Area(No.2009318802074),and West Project of Ministry Communication,China(No.2009318000074).
References
[1]Varnes D.J.,1978.Slope Movement Types and Processes[J].Transportation Research Board Special Report,1:176.
[2]Pistocchi A.,Lucia L.,Napolitano P.,2002.The Use of Predictive Modeling Techniques for Optimal Exploitation of Spatial Databases:A Case Study in Landslide Hazard Mapping with Expert System-like Methods[J].Environmental Geology,41(7):765-775.
[3]Yesilnacar E.,Hunter G.J.,2004.Application of Neural Networks for Landslide Susceptibility Mapping in Turkey[M].Netherlands:Kluwer Academic Publishers.
[4]Melchiorre C.,Matteucci M.,Azzoni A.,Zanchi A.,2008.Artificial Neural Networks and Cluster Analysis in Landslide Susceptibility Zonation[J].Geomorphology,94(3):379-400.
[5]Yin K.L.,Zhang G.R.,Gong R.X.,2005.Early-warning and Prediction of Abrupt Geological Hazards in Zhejiang Province[M].Beijing:China University of Geosciences Press.
[6]Dai F.C.,Lee C.F.,Ngai Y.Y.,2002.Landslide Risk Assessment and Management:an Overview[J].Engineering Geology,64(1):65-87.
[7]Zhou M.,Ren P.A.,1999.Fitting Degree of Regression Straight Line[J].Journal of Xi’an Engineering University,(3):74-76.
[8]Li W.L.,Cui J.K.,2002.On Parameter Estimation of the Largest Fittings[J].Journal of Shanxi Institute of Technology,18(4):46-48.
[9]Fang H.Y.,Chen J.J.,2001.BP Model for Hydrologic Series Prediction And Goodness of Fit Analysis[J].Journal of Yangzhou University,4(4):57-61.
[10]Li F.P.,Liu S.J.,1998.The Matter Element Analytical Method of the Assementof Rock Mass Stability[J].Gold,2:76-78.
[11]Zuo C.Q.,Chen J.P.,2007.Rock Mass Classification Based on Extenics Theory Appliedin Metamorphic Soft Rock Tunnel[J].Geological Science and Technology Information,3:15.
[12]Hao H.C.,Zhu F.H.,2007.Recognition Method of Potential Failure Mode of Slope Based on Extenics Theory[J].Chinese Journal of Underground Space and Engineering,4:24.
[13]Wang L.,1999.Topology Analysis for Steadiness of Side Slope of Rock Body[J].Hebei Metallurgy,1:21-23.
ICRE2016-International Conference on Railway Engineering